Share This Article
Overview
In the rapidly evolving landscape of AI technology, Meta CEO Mark Zuckerberg has offered a thought-provoking perspective on the significance of feedback loops in shaping the trajectory of AI development.
Amidst the AI arms race, characterized by the fervent pursuit of expansive data repositories, Zuckerberg has delineated a contrasting viewpoint on the essence of AI training. He stresses, “The thing that I think is going to be more valuable is the feedback loops rather than any kind of upfront corpus.”
Feedback loops, instrumental in refining AI models over time based on their past outputs, form the cornerstone of Zuckerberg’s elucidation. The integration of these algorithms allows AI to self-modify in response to errors and user interactions, presenting a pivotal shift from the traditional emphasis on amassing data resources.
The prominence accorded to feedback loops aligns with the evolving approach to enhancing AI capabilities. Instead of fixating solely on data acquisition, the emphasis is placed on continuous learning and adaptation, wherein AI systems garner insights from user interactions to evolve and improve their functionality.
The discourse surrounding data acquisition has assumed a fervent pace, with major tech entities resorting to diverse approaches in their quest for an expansive AI dataset. Treading unorthodox pathways, companies have explored acquisitions and even considered generating synthetic data as a means of satiating the data hunger of AI algorithms.
Utilizing synthetic data, i.e., artificially generated data designed to mimic real-world events, Zuckerberg underscores the potential of this approach. He suggests, “I think there’s going to be a lot in synthetic data, where you are having the models trying to churn on different problems and see which paths end up working, and then use that to reinforce.”
Amid these developments, the AI landscape presents a confluence of opportunities and risks. While the integration of feedback loops offers a promising avenue for AI progression, the potential for entrenching biases and limitations looms as a critical concern.
Zuckerberg’s perspective invites a broader conversation encompassing diverse viewpoints on the evolving dynamics of AI. It prompts deliberation on the multifaceted implications of prioritizing feedback loops over data accumulation, spanning considerations of innovation, ethical AI development, and the potential impact on user experiences and interactions.
In the realm of AI innovation, Zuckerberg’s insights introduce a compelling dimension, fueling discussions on recalibrating strategies to harness the transformative potential of feedback loops. Additionally, they underscore the imperative of aligning AI development with ethical frameworks and user-centric principles.
In essence, the dialogue spurred by Zuckerberg’s revelations draws attention to the evolving narrative of AI development, underscoring the need for a holistic perspective that acknowledges the interplay between data, feedback loops, and ethical considerations in sculpting the future trajectory of AI technology.
The Significance of Feedback Loops in AI Development
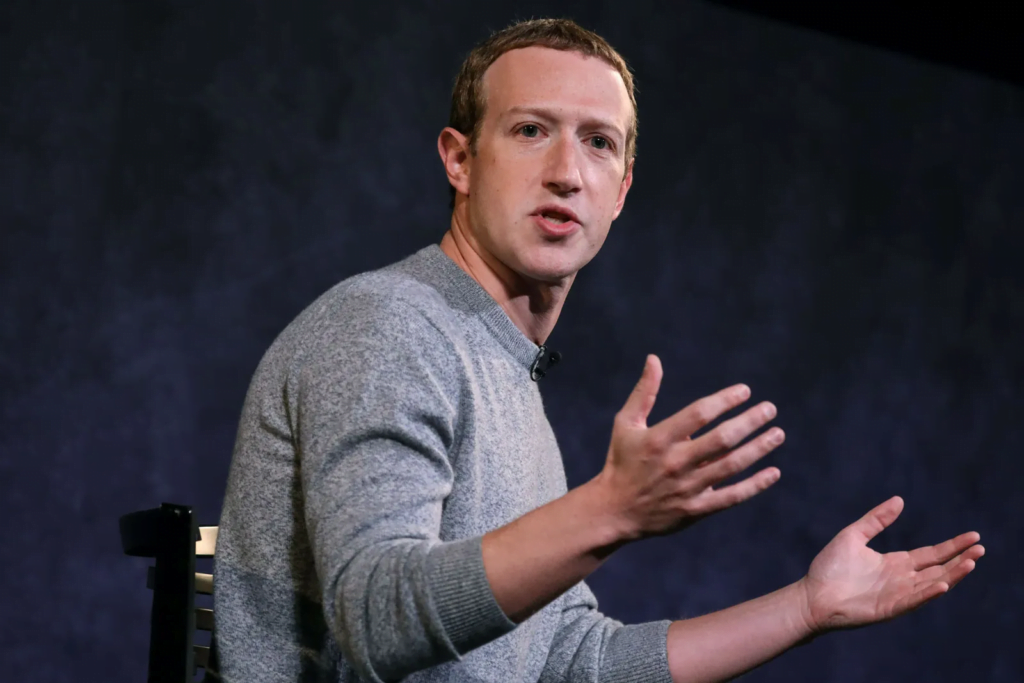
The traditional framework for AI development revolves around data accumulation, with companies vying to amass massive datasets to train their AI models. Data accumulation forms the bedrock for AI model panning, featuring inputs in varying modalities including video, audio, and text.
However, this approach exhibits certain limitations in the context of making AI efficient and effective. AI algorithms such as deep learning models are resource-intensive, with large amounts of data required to train them to attain optimal performance levels.
This approach is capital-intensive and requires substantial infrastructure investments, making it challenging for small-scale AI development teams to participate. Furthermore, the sheer magnitude of real-world data, spanning billions of user data points, makes it impractical to capture and analyze all information sources.
Feedback loops represent a significant paradigm shift in the AI development landscape. An AI system that leverages feedback loops can iterate continuously in response to real-world events, tweaking its functionality to adapt to changing requirements.
Zuckerberg’s perspective introduces a shift from the traditional ideology of data accumulation and resource-intensive model building. While data accumulation forms an essential ingredient to develop AI in its nascent stages, it has become imperative to consider methods that enable models to learn over time, iteratively adapting based on their performance.
This approach is more efficient and practical, enabling AI developers to focus on iterative improvements to their models, rather than constantly seeking to acquire more data. Feedback loops promote innovation and enable AI technology to evolve, improving its functionality and utility.
The Role of Synthetic Data in AI Development
As the demand for AI and ML solutions continues to rise, so does the need for vast datasets to train algorithms and refine their accuracy. However, obtaining such data can be problematic, particularly for small-scale AI development teams and organizations.
Synthetic data is a novel approach trending in the AI landscape. The use of artificially generated data to expose machine learning systems to real-world scenarios drives innovation and promotes the development of more efficient AI systems.
Synthetic data is typically generated through algorithms and AI models designed to simulate real-world events such as traffic scenarios, medical samples, and other scenarios. The resultant dataset is integrated into the AI system and enables it to learn and improve.
According to Zuckerberg, his use case for synthetic data is multi-dimensional, ranging from training deep learning models to validating the performance of an AI algorithm, particularly in tackling specific problems. This approach saves costs and resources while still exposing AI systems to real-world scenarios.
However, the use of synthetic data still represents a significant challenge, as the resultant dataset may not accurately capture all data inputs or nuances present in the real world. Furthermore, synthetic data can also exhibit biases and limitations, potentially restricting the accuracy and effectiveness of AI models.
The Importance of Ethical and User-Centric AI Development
As AI technology makes headway into critical domains such as healthcare, finance, and transportation, it is pivotal to consider its ethical implications. AI systems are subject to various errors, biases, and limitations inherent in the data fed to them. These issues raise concerns about whether AI systems are robust and safe, particularly when applied to critical functions.
Zuckerberg’s emphasis on feedback loops brings ethical considerations to the forefront of the AI development discourse. The integration of feedback loops can mitigate errors and biases, promoting fair and equitable AI technology.
For instance, recent studies have demonstrated that AI systems can possess racial and gender biases, impacting their output and influencing decision-making processes. Feedback loops allow artificial intelligence algorithms to self-modify and account for these biases over time, ultimately promoting fairness and inclusivity.
Moreover, AI development should aim to cater to user needs and requirements, ensuring that user experiences remain at the forefront of AI development. This aspect emphasizes the need for an integrated approach to AI development, encapsulating ethics, user-centric approaches, and technical considerations.